To get into the topic, it is useful to have some basic knowledge about graphs first. A knowledge graph is a structured representation of information that is presented in the form of entities (objects, people, or concepts) and their relationships to each other. The knowledge graph receives the data from a database, for example Wikidata, as an open source, news agencies, social media or editorial databases. If these are then displayed in a knowledge graph, you get the familiar blackboard image from countless crime films. All the people on the board are connected by red threads, making their relationships and networks visible, but the knowledge graph takes this to a new level with thousands of links and the ability to apply further algorithms to them. Basically a 3D blackboard with a built-in search.

A practical example from the editorial environment
I am writing a current story about new research on a meeting of several people in Potsdam who may not have been in contact with each other before. The Knowledge Graph, fed from a database, can search out all the links between the two people within seconds and thus perhaps provide research approaches for a multidimensionality of the story. Perhaps the two people were enrolled at the same university or had already met on another occasion?
A knowledge graph can provide knowledge that an editor has to learn and for which a great deal of learning effort is required for knowledge transfer in the editorial department in a very short time.
There is a risk that individual connections may remain undiscovered or be forgotten. The knowledge graph facilitates this transfer, it can make knowledge about objects, people, and entities easily transferable, and not just for people.
Large language models (LLM) such as ChatGPT or Bard can provide incorrect information or hallucinate.
They may be able to handle the language and context, but the underlying information is misleading or the facts are made up by the LLM. In an environment where we thrive on accurate information and where it is a top priority, we need reliability. And that is exactly what the combination of an LLM that bases its information on a knowledge graph provides.
Whereas LLM training requires an extremely large number of texts as training material in order to create language comprehension at all, the knowledge graph can be used to learn the connections between different pieces of information and the verified facts, free from trained fuzziness or errors.
KNOWLEDGE GRAPH
A knowledge graph connects knowledge by representing information in the form of points (entities such as objects or people) and linking them with lines (relationships). The points represent individual elements of knowledge, while the lines show the relationships between them.
But this is just the beginning: processing in a knowledge graph offers further analysis options.
Machine learning makes other correlations visible that are difficult to access for human analysis. For example: “Which actresses under 1.60 cm have won an Oscar?” A research that would be more time-consuming without the database, but thanks to the graph only takes a few seconds.
The combination of knowledge graphs and LLMs enables the creation of specialized LLMs that not only master language, but can also generate precise information in any subject area – indispensable in future-proof companies or editorial offices.
This reliability is particularly important in light of upcoming regulatory requirements such as the EU’s Artificial Intelligence Act. Transparent and traceable LLMs based on knowledge graphs are better able to fulfill the prescribed rules, as they are more trustworthy and 100% traceable due to their contextual and verified data.
How can such a knowledge graph find its way into journalistic processes – a use case with CGI’s Open Media.
CGI OpenMedia meets DeepVA
The CGI OpenMedia Newsroom Computer System (NRCS) acts as a collaborative platform for journalists, providing the information, functionality, and transparency needed to meet the challenges of daily news production. It ensures maximum speed and scalability for journalistic workflows in broadcast news production, from wire search to scripting to playout control.
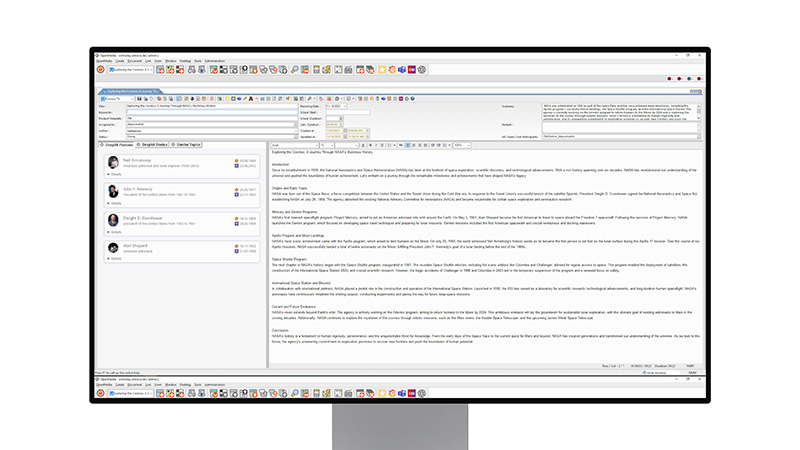
DeepVA’s integration with OpenMedia enables the seamless use of DeepVA’s intelligent features without the need to manage multiple applications. Journalists can quickly and easily obtain relevant information to support, improve and accelerate their daily research. DeepVA identifies people in the body text of an OpenMedia story and displays them along with interesting personal data such as a short description, date of birth and possible date of death. It also suggests similar OpenMedia stories and lists them in the current story with important details, allowing the user to automatically access all the details.